What Is AI?
Learn about AI
Explore how to get the best out of it, ensuring responsible use.
Artificial Intelligence (AI) is a discipline that enables machines to mimic intelligent human behavior.
Artificial General Intelligence (AGI) refers to the ability of a machine to perform any task a human being is capable of.
Current capabilities can be positioned along what is called the âjagged technological frontierâ.
AI and Generative AI are changing the way people learn, work and live â there are opportunities but also challenges.
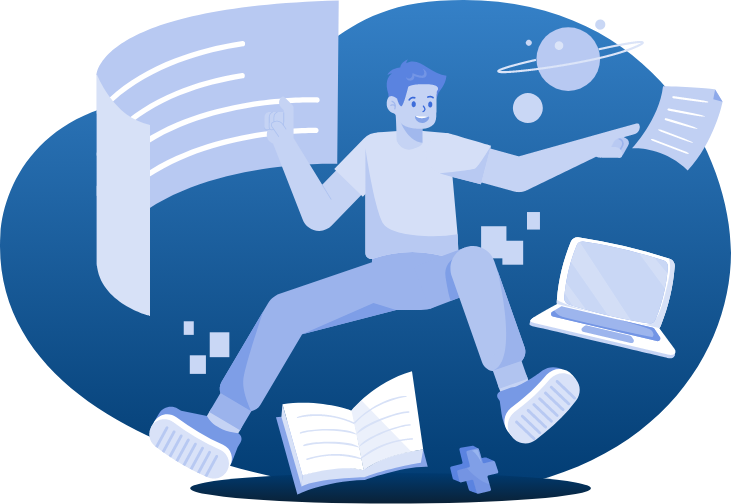
These capabilities of AI to produce human-like work have improved rapidly, especially since the release of Generative AI, a category of Machine Learning technology that is able to produce new multimedia content based on existing data patterns.
AI Basics
Programs that can find patterns or make decisions from a previously unseen dataset. They are built on foundations of algorithms and large datasets that enable them to analyze patterns and solve complex problems
Feedstock of AI. Feed an AI model text-image pairs and the AI model develops the ability to recognize or generate images. Feed a GenAI model music and it will compose music. âYou are what you eatâ perfectly captures the importance of data as an AI building block.
Computational resources and processing power required to train, develop and run AI models. These resources include central processing units (CPUs), graphics processing units (GPUs) and specialized hardware like tensor processing units (TPUs).
Some definitions
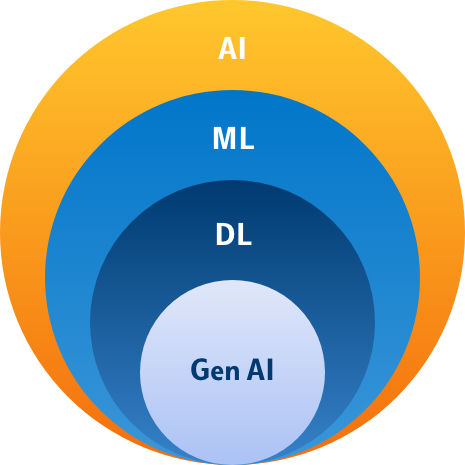
Artificial intelligence is a technical and scientific field devoted to the engineered system that generates outputs such as content, forecasts, recommendations or decisions for a given set of human-defined objectives. [ISO/IEC 22989:2022]
A subset of AI that uses algorithms to learn insights and recognize patterns from data, applying it to make better decisions. ML can be supervised (i.e., using labeled datasets) or unsupervised (i.e., finding patterns within unlabeled datasets).
Advanced subset of Machine Learning that leverages neural networks to learn from vast amounts of data.Neural networks enable systems to learn hidden patterns from data by themselves and build more efficient decision rules.
Popular applications of DL include computer vision and natural language processing (NLP).
A subset of artificial intelligence that uses generative models to produce text, images, videos, or other forms of data. These models learn the underlying patterns and structures of their training data and use them to produce new data based on the input, which often comes in the form of natural language prompts.
AI Capabilities
AI capabilities cover an expanding, but uneven, set of knowledge work that has been represented by the âjagged technological frontierâ. This is a âvirtualâ line, moving through time as technology advances, and separating tasks âinside the frontierâ from tasks âoutside the frontierâ.
The rationale is to distinguish what is within this moving frontier, i.e., tasks in which AI technologies can complement or even outperform human work, from what is outside the frontier, where the output produced by AI technologies can be inaccurate, less useful, or degrades human performance.
People who skillfully navigate this frontier gain large productivity benefits when working with AI technologies, while AI technologies can actually decrease performance when used outside the frontier.As the capabilities of AI are rapidly evolving and poorly understood, it can be hard for professionals to grasp exactly what the boundary of this frontier might be at a given point.
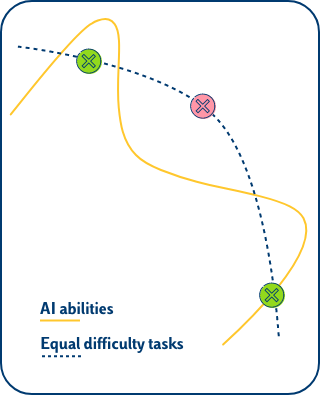
Generative AI
Two discoveries have made the history of Generative AI: the transformer and the related attention mechanism.
Generative AI tools are input-outputs machines. The input is given by the user and with the new Multimodal AI can be in different formats (text, image, video, audio, code).
The output is different depending on the specific tool, and can be text, image, video, audio, code as well.
Most LLMs have in them an element of randomness that allows them to return slightly different results each time they are asked the same question.
Moreover, their functioning can be set according to temperature which represents the accuracy in generating the new information, compared with the training/input data.
Prompting
Generative AI systems produce outputs based on prompt quality, and prompt engineering improves their ability to handle various queries effectively.
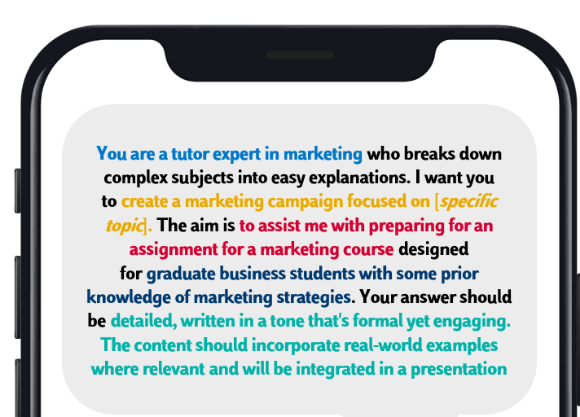
The elements of a good prompt:
Persona: ask the tool to take a role.
Objective: define what you want the AI to do.
Audience: specify who the output of the prompt is for.
Context: indicate what the tool needs to know.
Boundaries: set your own direction & limitation.
Tip: iterate and engage in a conversation, trying to spot pitfalls in your requests and crafting different prompts on the same task. In this way, you will critically evaluate the output of the LLM but you will also practice your metacognitive skills (learning how to think about something and evaluating your reflection processes).
AI Challenges
Artificial intelligence (AI) is revolutionizing various fields, enhancing innovation and efficiency. However, its rapid advancement also brings significant challenges that require careful consideration.
AI models may perpetuate existing biases found in their training data, impacting outcomes, and consequently, decisions informed by them.
Providing personal or sensitive data to AI systems is risky in terms of privacy, due to difficulties in tracing their use and transfer.
Known as the âBlack Boxâ problem, AI often lacks transparency in how it processes inputs and produces outcomes.
AI use in academic research and content creation raises concerns about unintentional plagiarism.
Excessive use of AI may reduce creativity and novelty in solutions and ideas.
Over-reliance on AI may produce a de-skilling of human competencies; it can be detrimental toour ability to learn, critically analise and independently solve tasks.
Key issues in academia
- Academic integrity: risk of plagiarism or counterfeit assessments, essays, and exams.
- Over-monitoring: effectively limiting or preventing AI misuse by students can become very time-consuming and stressful for instructors.
- Hallucinations:Â AI outputs can be unreliable and provide plausible but incorrect information, due to the statistical recombination of input data.
- Misuse of AI: AI may be used for purposes beyond its intended aim, leading to unethical or illegal applications.
- Innovate teaching and evaluations: AI can foster the design of personalized activities and assessments, promoting a shift towards active learning and critical engagement with course materials.
- Interactive Learning: AI tools can facilitate interactions, via simulations, debates, and real-time feedback, improving comprehension and engagement.
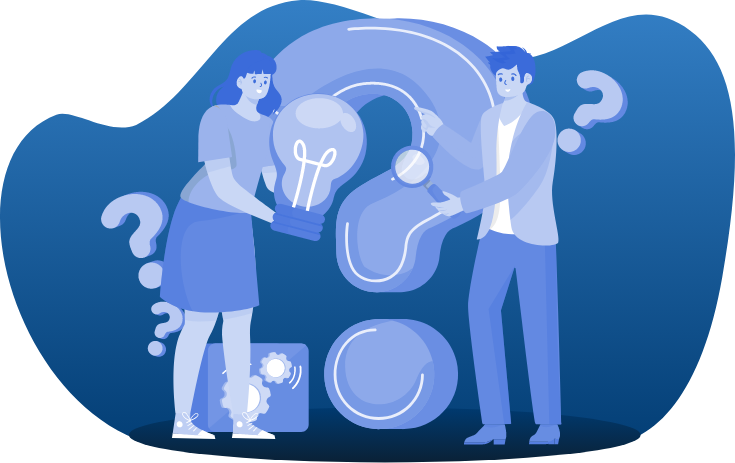
The Latest Research on AI
In the article âThe case for humanâAI interaction as system 0 thinkingâ by Chiriatti et al. (2024), published in Nature Human Behaviour, the concept of âsystem 0â was introducedâa cognitive framework in which AI enhances human thinking by processing vast amounts of data and performing complex calculations.
This system interacts with intuitive and analytical thinking, raising important epistemological and ethical questions.
Can generative artificial intelligence facilitate or hinder learning? This is the central question of an article by Bastani et al. (2024), based on a study conducted in high school math classes, evaluating the effects of OpenAI’s GPT-4 on student learning.
It should be noted that the issue of the effects of AI on learning is widely debated, so this source should not be taken as the only evidence on the phenomenon.
The 2024 study by Pinski and Benlian examines research on AI literacy, providing a framework of needed skills and an agenda to help users engage with AI technologies effectively and ethically.